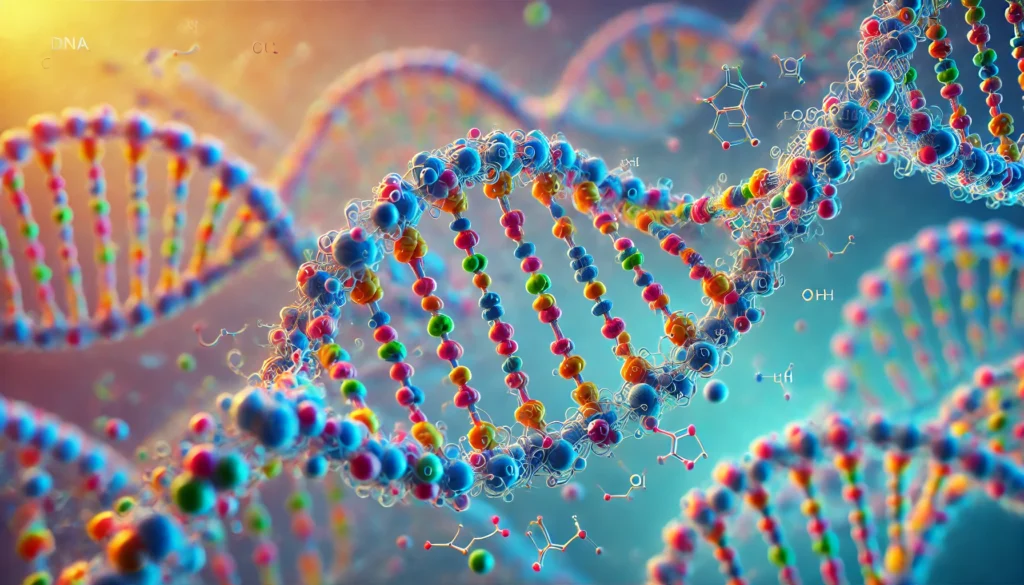
The field of molecular design is undergoing a seismic shift thanks to advancements in artificial intelligence (AI). Imagine a world where designing life-saving drugs, creating sustainable materials, or even solving complex biological problems is as streamlined as writing a piece of code. That’s not just a dream—it’s the transformative power of AI-driven molecular design.
This blog delves into what AI-driven molecular design is, its groundbreaking applications, challenges, and what lies ahead. Whether you’re a curious beginner or a seasoned scientist, we’ll guide you through this exciting field in an approachable, engaging, and fun way. Let’s dive in!
What is AI-Driven Molecular Design?
At its core, molecular design involves creating and optimizing molecules for specific purposes, like curing diseases or developing advanced materials. Traditional approaches to molecular design have relied heavily on trial and error, guided by human intuition and time-consuming experimentation. Enter AI: the ultimate game-changer.
AI-driven molecular design leverages powerful algorithms, particularly machine learning (ML) and deep learning, to accelerate and enhance this process. By analyzing vast datasets of molecular structures and properties, AI models can predict the behavior of new molecules, suggest innovative designs, and even simulate their interactions—all in record time.
Think of AI as the chef in a molecular kitchen: it knows all the ingredients (atoms), understands the recipes (chemical reactions), and can whip up a five-star molecular dish faster than ever before.
How Does AI Work in Molecular Design?
To appreciate the magic of AI in this field, let’s break it down step by step:
1. Data is the Foundation
AI thrives on data. Molecular design requires vast datasets of molecules, their structures, and corresponding properties, such as solubility, stability, or biological activity. These datasets are like textbooks that teach AI how molecules behave in different scenarios.
2. Training AI Models
Once the data is collected, AI models are trained to recognize patterns and make predictions. For example:
- Classification Models: Determine whether a molecule is likely to be toxic.
- Generative Models: Propose entirely new molecular structures based on desired characteristics.
- Predictive Models: Forecast how a molecule might interact with a target, such as a protein in the human body.
3. Molecular Simulation
AI doesn’t stop at suggestions—it simulates how molecules behave. Techniques like quantum mechanics and molecular dynamics are computationally expensive, but AI streamlines these processes, making simulations faster and more accurate.
4. Optimization and Iteration
AI continuously improves designs by iterating. If a molecule isn’t performing as desired, AI tweaks it, learning from every step, much like an artist refining a masterpiece.
Applications of AI-Driven Molecular Design
From healthcare to sustainability, the applications of AI-driven molecular design are as vast as they are impactful. Here’s a look at some of the most exciting areas:
1. Drug Discovery
Developing a new drug traditionally takes over a decade and billions of dollars. AI is slashing these numbers dramatically. Here’s how:
- Identifying Drug Targets: AI can analyze biological data to pinpoint disease-causing proteins or pathways.
- Designing Drug Molecules: Once a target is identified, AI generates molecules likely to bind to it effectively, a process that previously required years of work.
- Predicting Side Effects: AI can foresee potential side effects by analyzing molecular interactions, reducing risks during clinical trials.
Fun Fact: During the COVID-19 pandemic, AI tools helped identify potential antiviral compounds in record time, highlighting its power in urgent scenarios.
2. Material Science
Beyond medicine, AI is revolutionizing material design. Need a super-strong, lightweight material for aerospace engineering? Or a biodegradable plastic? AI can help:
- Discovering Novel Materials: AI generates molecular structures optimized for specific mechanical, thermal, or electrical properties.
- Accelerating Sustainability: By designing materials that are recyclable or derived from renewable resources, AI contributes to environmental conservation.
3. Personalized Medicine
AI-driven molecular design is paving the way for personalized medicine, tailoring treatments to an individual’s unique genetic makeup. By designing molecules that work specifically for a patient’s biological profile, AI is making “one-size-fits-all” treatments a thing of the past.
4. Agricultural Innovation
In agriculture, AI is helping design molecules like fertilizers, pesticides, and herbicides that are more effective and environmentally friendly. This means higher crop yields with fewer ecological repercussions—a win-win for farmers and the planet.
5. Tackling Climate Change
AI is being used to design carbon capture materials and optimize processes that reduce greenhouse gas emissions. For instance:
- Catalyst Design: AI accelerates the discovery of catalysts that convert carbon dioxide into useful products.
- Energy Storage: AI helps create better batteries and fuel cells, key to renewable energy systems.
The AI Toolkit for Molecular Design
Let’s take a quick peek at some of the specific AI tools and techniques used in molecular design:
1. Neural Networks
Deep learning models, particularly neural networks, are the backbone of many AI-driven molecular design systems. These models excel at recognizing complex patterns in molecular data.
2. Generative Adversarial Networks (GANs)
GANs are like molecular idea generators. They pit two neural networks against each other: one creates new molecules, and the other critiques them, leading to ever-improving designs.
3. Reinforcement Learning
Reinforcement learning models learn by trial and error. They experiment with molecular designs, receive feedback, and refine their approach to achieve optimal results.
4. Quantum Computing
While still in its infancy, quantum computing holds immense promise for molecular design, allowing AI to solve problems too complex for classical computers.
Challenges in AI-Driven Molecular Design
Despite its promise, AI-driven molecular design isn’t without hurdles. Here are some challenges and how the field is tackling them:
1. Data Quality and Availability
AI models are only as good as the data they’re trained on. High-quality, diverse molecular datasets are essential, but collecting and curating such data is no small feat.
Solution: Collaborative initiatives and open databases are helping address this issue. For example, platforms like the Protein Data Bank provide valuable resources for researchers.
2. Computational Costs
Simulating molecular interactions or training complex AI models can be computationally expensive.
Solution: Advancements in hardware, such as GPUs and TPUs, along with more efficient algorithms, are making these processes faster and cheaper.
3. Interpretability
AI models are often criticized as “black boxes,” making it hard to understand why they make certain predictions.
Solution: Efforts are underway to develop explainable AI systems that provide insights into their decision-making processes.
4. Ethical Concerns
AI-driven molecular design raises ethical questions, such as the potential misuse of the technology in designing harmful substances.
Solution: Strict regulations, transparency, and ethical oversight are critical to ensuring AI is used responsibly.
The Future of AI-Driven Molecular Design
What’s next for this game-changing field? Here are some exciting possibilities:
- AI-Powered Labs: Fully automated laboratories where AI designs, synthesizes, and tests molecules, dramatically accelerating research.
- Real-Time Drug Development: Imagine diagnosing a disease and designing a personalized treatment in a matter of hours, all thanks to AI.
- Cross-Disciplinary Synergies: Combining AI-driven molecular design with fields like robotics, nanotechnology, and synthetic biology to tackle challenges we can’t yet imagine.
Why Should You Care?
AI-driven molecular design isn’t just for scientists in lab coats. Its impact ripples through every aspect of our lives:
- Better Healthcare: Faster cures and fewer side effects.
- A Greener Planet: Sustainable materials and eco-friendly innovations.
- Economic Growth: Reduced costs and faster product development.
In short, AI-driven molecular design is shaping a brighter, healthier, and more sustainable future.
Conclusion
AI-driven molecular design represents the perfect blend of science, technology, and creativity. By harnessing the power of AI, we’re transforming the way we approach complex problems, opening doors to discoveries once thought impossible.
Whether it’s a life-saving drug, a revolutionary material, or a solution to climate change, the molecules of tomorrow are being designed today—by AI. The future has never looked so promising, or so molecularly precise!
So, next time you hear about AI, remember: it’s not just about chatbots or self-driving cars. It’s designing the building blocks of our universe—and doing it better, faster, and smarter than ever before. Welcome to the molecular revolution!